AI for Global Health and Public Health
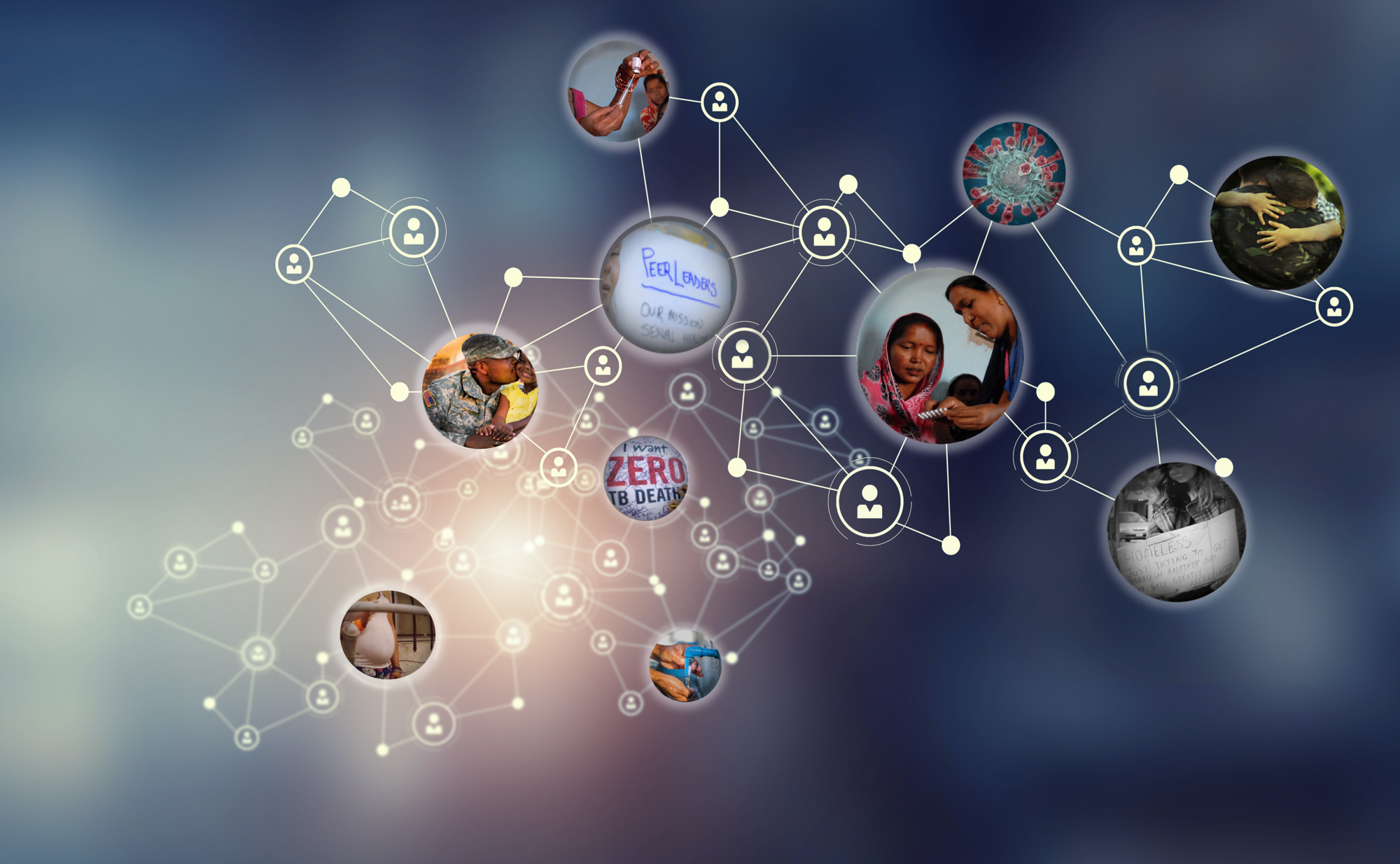
Modeling to Inform Disease Control, Screening, Treatment Policies, and Prevention Interventions
MOTIVATION
CURRENT PROJECTS
Improving Maternal and
Child Health Outcomes in
Partnership with ARMMAN
This project Improving Maternal and Child Health Outcomes in Partnership with ARMMAN aims at using AI for improving Maternal and Child Health Outcomes by predicting which beneficiaries are at a risk of dropping out from automated health information delivery programs.
Learning Loss Functions for Predict-then-Optimize
HIV Prevention
Among Homeless Youth
The HIV Prevention Among Homeless Youth project focuses on the development of decision support systems for homeless youth drop-in center staff, who need to find the most influential homeless youth to raise awareness about HIV (and other STDs) among their peers, and to drive the homeless youth community towards safer behaviors.
PREVIOUS WORK
Algorithmic Social Interventions for Social Work and Public Health
Combatting COVID-19
The COVID-19 outbreak has caused an unprecedented global reaction with countries taking drastic steps to combat the pandemic. Mathematical modeling and multi-agent based analysis of the pandemic allows better understanding of the disease spread and may help inform policy at the national and regional level. We use tools and modeling techniques from AI to help understand the situation better and design aids that may help policymakers design better solutions in the fight against this pandemic. Read more about Teamcore's efforts to stop the spread of COVID-19.
Using Machine Learning
& Multi-Agent Planning
to Fight Tuberculosis

Fighting and Preventing Tuberculosis in India
Tuberculosis is one of the top 10 killers in the world and is especially prevalent in India. AI can help across the entire pipeline of care, from decision support tools for planning active screening routes, to predictive algorithms for resource constrained health workers to deliver targeted interventions to patients.
Using Social Networks
for Prevention Interventions
Click here to read more on this initiative.
Substance abuse prevention among homeless youth
Research has consistently documented levels of cocaine, heroin, methamphetamine, alcohol, and marijuana use and abuse among these adolescents that far exceed that of housed adolescents. This Social Networks and Substance Abuse Prevention for Homeless Youth project aims to use algorithms to determine the best group formations to prevent regular use of hard drugs among homeless youth.
Suicide prevention among active duty military and homeless youth
One of the fundamental questions facing social science is how social networks and the cognitions people have about their networks affect their mental states and mental health. AI techniques present an opportunity to dynamically model social networks and the messages transmitted across those networks to create predictive models of influence unavailable with standard statistical techniques. View Predictive Modeling for Early Identification of Suicidal Thinking.
PROJECT
PARTICIPANTS
Sze-chuan Suen
Milind Tambe
Bryan Wilder
Han Ching Ou
Dana Goldman
Eric Rice
Carl Castro
Anthony Fulginiti
Anamika Barman-Adhikari
Aditya Mate
Phebe Vayanos
Aida Rahmattalabi
Jackson A. Killian
Maimuna Majumder
Marie Charpignon
Angel N. Desai
Shahin Jabbari
Andrew Perrault
Lily Xu
Shresth Verma
Aparna Taneja
Vineet Nair
Aparna Hegde
Neha Madhiwalla
Paula Rodriguez Diaz
Sonja Johnson-Yu
Sanket Shah
Become a Harvard Teamcore partner through community projects, funding, volunteering, or research collaboration.